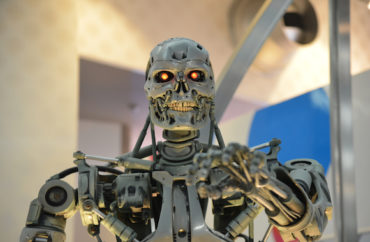
‘Flawed algorithms can amplify biases through feedback loops’
Two professors from Stanford University argued recently in an article that A.I., or artificial intelligence, can be “sexist and racist” and that the technology community needs to implement “systematic solutions” to counteract this threat.
James Zou and Londa Schiebinger note in an article on Stanford’s website that, in some instances, Google Translate can change pronouns from female to male. As well, “Software designed to warn people using Nikon cameras when the person they are photographing seems to be blinking tends to interpret Asians as always blinking.” Additionally, “Word embedding, a popular algorithm used to process and analyse large amounts of natural-language data, characterizes European American names as pleasant and African American ones as unpleasant.”
“These are just a few of the many examples uncovered so far of artificial intelligence (AI) applications systematically discriminating against specific populations,” the instructors write.
A large part of the problem, the professors argue, is in the “training data” used to build artificial intelligence. This data, they write, which is often drawn from “large, annotated data sets” such as Wikipedia and Google News, “can unintentionally produce data that encode gender, ethnic and cultural biases.”
From the article:
Frequently, some groups are over-represented and others are under-represented. More than 45% of ImageNet data, which fuels research in computer vision, comes from the United States2, home to only 4% of the world’s population. By contrast, China and India together contribute just 3% of ImageNet data, even though these countries represent 36% of the world’s population. This lack of geodiversity partly explains why computer vision algorithms label a photograph of a traditional US bride dressed in white as ‘bride’, ‘dress’, ‘woman’, ‘wedding’, but a photograph of a North Indian bride as ‘performance art’ and ‘costume’2.
In medicine, machine-learning predictors can be particularly vulnerable to biased training sets, because medical data are especially costly to produce and label. Last year, researchers used deep learning to identify skin cancer from photographs. They trained their model on a data set of 129,450 images, 60% of which were scraped from Google Images3. But fewer than 5% of these images are of dark-skinned individuals, and the algorithm wasn’t tested on dark-skinned people. Thus the performance of the classifier could vary substantially across different populations.
“[T]echnical care and social awareness must be brought to the building of data sets for training,” the professors write. “Specifically, steps should be taken to ensure that such data sets are diverse and do not under represent particular groups.”
MORE: Law professor warns: Congress must act on growing sexbot industry
IMAGE: Sarunyu L / Shutterstock.com
Please join the conversation about our stories on Facebook, Twitter, Instagram, Reddit, MeWe, Rumble, Gab, Minds and Gettr.